Table Of Content
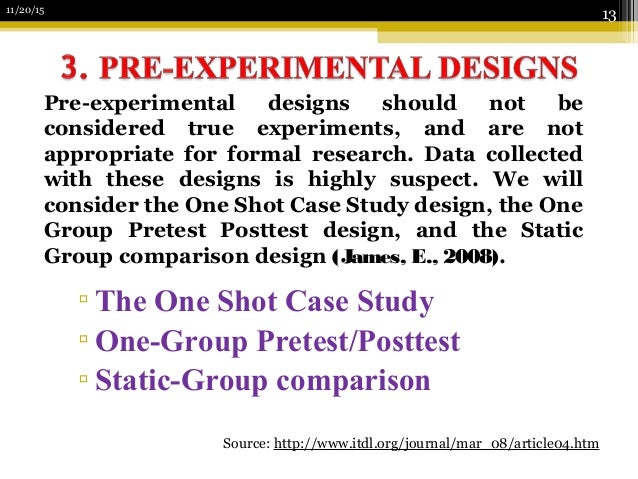
Pre-experimental designs, thus, are usually conducted as a first step towards establishing the evidence for or against an intervention. However, this type of design comes with some unique disadvantages, which we’ll describe as we review the pre-experimental designs available. Though it is beyond the scope of this textbook to describe the plethora of quasi-experimental designs, one more design is worth mentioning.
Lack of Control Group
In the static group comparison study, two groups are chosen, one of which receives the treatment and the other does not. A posttest score is then determined to measure the difference, after treatment, between the two groups. As you can see, this study does not include any pre-testing and therefore any difference between the two groups prior to the study are unknown. The authors of many of these guidelines state that their list might need adaption to the specific experiment. This is pointing out the general shortcoming that a fixed-item list can hardly foresee and account for any possible experimental situation and a blind ticking of boxes ticking of boxes is unlikely to improve experimental design.
Types of Pre-experimental Designs
There is also a risk of selection bias, and the findings may not be generalizable to other populations or settings. There are several types of pre-experimental designs, including the one-shot case study, the one-group pretest-posttest design, and the static-group comparison. As a result, it’s difficult to determine whether observed changes are the result of the intervention or due to extraneous factors. Pre-experimental designs are characterized by their simplicity and ease of execution.
Table 5.1: Diagrams of Pre-Experimental Designs
As you might have guessed from our example, static group comparisons are useful in cases where a researcher cannot control or predict whether, when, or how the stimulus is administered, as in the case of natural disasters. Unfortunately, it is difficult to know those groups are truly comparable because the experimental and control groups were determined by factors other than random assignment. Quasi-experimental designs are similar to true experiments, but they lack random assignment to experimental and control groups. The most basic of these quasi-experimental designs is the nonequivalent comparison groups design (Rubin & Babbie, 2017). [1] The nonequivalent comparison group design resembles the classic experimental design, but it does not use random assignment.
If we wished to measure the impact of a natural disaster, such as Hurricane Katrina for example, we might conduct a pre-experiment by identifying a community that was hit by the hurricane and then measuring the levels of stress in the community. Researchers using this design must be extremely cautious about making claims regarding the effect of the treatment or stimulus. They have no idea what the levels of stress in the community were before the hurricane hit nor can they compare the stress levels to a community that was not affected by the hurricane.
(PDF) "A study to assess the effectiveness of structured teaching programme on knowledge of mothers regarding ... - ResearchGate
(PDF) "A study to assess the effectiveness of structured teaching programme on knowledge of mothers regarding ....
Posted: Thu, 19 Oct 2023 04:49:52 GMT [source]
These designs are called “pre-experimental” because they precede true experimental design in terms of complexity and rigor. The true experimental design carries out the pre-test and post-test on both the treatment group as well as a control group. It does not always have the presence of those two and helps the researcher determine how the real experiment is going to happen. As pre-experimental design generally does not have any comparison groups to compete for the results with, that makes it pretty obvious for the researchers to go through the trouble of believing its results. Because there is a chance that the result occurred due to some uncalled changes in the treatment, maturation of the group, or is it just sheer chance. This design attempts to make up for the lack of a control group but falls short in relation to showing if a change has occurred.
General Scientific Methods for Designing In Vivo Experiments
Assessments of research outputs should focus more on these factors and less on any apparent “novelty”. Even when pre-experimental designs identify a comparison group, it is still difficult to dismiss rival hypotheses for the observed change. This is because there is no formal way to determine whether the two groups would have been the same if it had not been for the treatment. If the treatment group and the comparison group differ after the treatment, this might be a reflection of differences in the initial recruitment to the groups or differential mortality in the experiment. The central challenge of the propensity score procedure is ensuring that the groups have been closely balanced on all important covariates. For example, matching on a few demographic variables is unlikely to represent fully all of the important baseline differences between the groups (Rubin, 2006).
First, some other confounding event (e.g., introduction of a new heart medication) may occur at about the same time as the introduction of the intervention. For example, some cities have offered college scholarships to all students who graduate from high school. In such cases, in addition to any effect of the program on the achievement of city residents, the introduction of the program may foster immigration of highly education-oriented families to the city, changing the nature of the student population. For example, new criteria for the diagnosis of angina or myocardial infarction may change the number of heart disease cases even in the absence of any effect of the intervention.
In the case of fuzzy RD designs, sensitivity analyses in which different plausible assumptions are made about alternative functional forms of the relationship and selection models can also be conducted. To understand the RD design, consider the example of evaluating the effectiveness of school lunch programs on health, which is illustrated in Figure E-1. In the figure, all children with a family income of less than $20,000 qualify for the program, whereas children whose families exceed this threshold do not. The outcome measure (here a measure of health problems, such as number of school absences or school nurse visits) is collected for each child. In modeling the relationship between the known quantitative assignment variable (family income) and the outcome, the treatment effect will be represented by the difference in the levels of the regression lines at the cutpoint. In the basic RD design, treatment assignment is determined entirely by the assignment variable.
These alternatives to evidence-based practice of the most literal and rigorous scientific variety suggest some advantages and complementarities of a model of practice-based evidence that produces locally adapted and prospectively tested evidence. Users of this and other guidance for linking research to the decisions they must make in their own settings will need to trade off some degree of rigor for more reality in the setting, conditions of practice, and free-living populations observed. To illustrate this design, consider an evaluation of a campaign to increase sales of lottery tickets (Reynolds and West, 1987). State lottery tickets are sold primarily in convenience stores and contribute to general state revenue or revenue for targeted programs (e.g., education) in several states. The Arizona lottery commission wished to evaluate the effectiveness of a sales campaign to increase lottery ticket sales in an 8-week-long lottery game. In the “Ask for the Sale” campaign, store clerks were instructed to ask each adult customer during checkout if he or she wished to purchase a lottery ticket.
Incidence of HIV disclosure among HIV affected heterosexual partners using a community health worker led ... - BMC Infectious Diseases
Incidence of HIV disclosure among HIV affected heterosexual partners using a community health worker led ....
Posted: Thu, 11 May 2023 07:00:00 GMT [source]
Therefore, researchers must exercise extreme caution in interpreting and generalizing the results from pre-experimental studies. Much of the published evidence in obesity-related research is epidemiological or observational, linking the intermediate causes or risk factors with obesity-related health outcomes. Therefore, the range of values of moderating variables (demographic, socio economic, and other social factors that modify the observed relationships) in models that predict outcomes is limited. Because of these limitations of the evidence-based practice literature, decision makers must turn to practice-based evidence and ways of pooling evidence from various extant or emerging programs and practices.
No comments:
Post a Comment